Recently, we’ve had the opportunity to try to tackle one of the real world problems the public sector faces today with some of our partners: communications during complex, high-stress situations with limited to no connectivity. We’re looking into LLMs (Large Language Models) as a potential solution for both smaller scales of operation (e.g. localised building fires, bomb threats) to the extremes (e.g. disaster relief in major cities, refugee protection in no man’s land) to alleviate communication friction, improve information efficiency and shorten response times by hours – crucial at times when lives hang on seconds.
We thought it’d be interesting to talk about some of the issues we’re looking to tackle, our philosophy and approach to them, and to highlight some of the challenges and learnings we’ve discovered along the way.
Problem Statements
Imagine this: A 7.5 earthquake hits Japan, followed by a tsunami. Hundreds of thousands of buildings and roads have collapsed, leaving many critically injured and requiring medical aid or rescue. Critical communications infrastructure have been damaged, leaving blank spots in network and radio connectivity, and mobile/satellite networks have been overloaded by a surge of public traffic. Survivors have no access to clean water, and 90% of power grids are down – not only in one town, but everywhere along the coast – leading to potential food shortages in the rest of the country. You’re a leader in the recovery effort – what do you do?
This is the 2024 Noto Peninsula Earthquake. 401 lives were directly lost, millions more were impacted, USD 17.6 billion has been estimated in damages, and recovery efforts are still ongoing today. One of the major challenges in disaster response includes the disruption of communications and coordination efforts, especially for first responders in the aftermath – this is our field of interest and the gap we’re attempting to bridge.
Some key issues we’ve identified that obstruct communications during disasters:
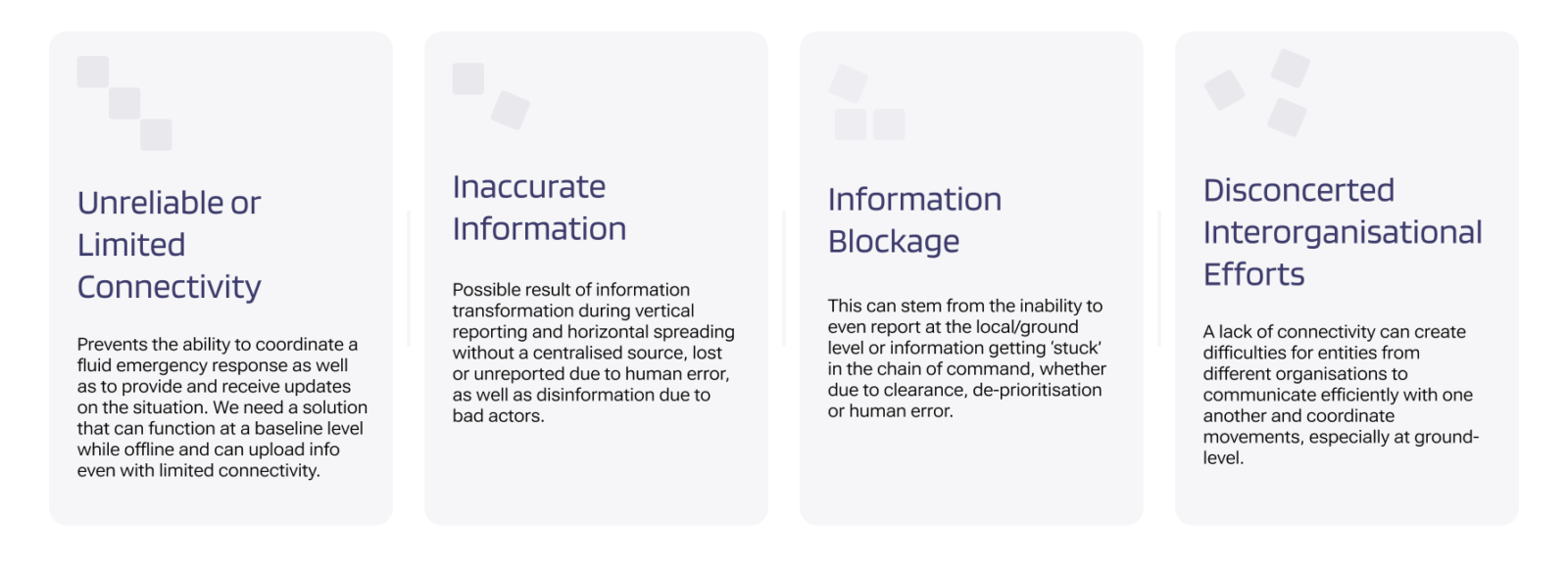
Our Approach
We’ve explored several ideas, but we’ve found the concept of democratising communication during a crisis to have the most promise. Units on the field need to have agency and access to information ASAP to provide a rapid response in a localised sphere. This might include things such as local maps, SOS signals, information from local informants, and reports generated by and accessible to ground-level users, as well as crowdsourced from the World Wide Web (e.g. 2015 Nepal Earthquake).
To achieve this, we’re proposing the development of an online/offline hybrid consolidated information environment that is shared across all units and entities and accessible from a mobile device. This information database would be supported by an LLM to handle queries, provide data, assimilate reports, and return information, even while offline. Whenever the device ventures back into a network zone or connectivity is turned back on, the local AI database is then updated with priority information from the main database while simultaneously returning any local updates from the ground.
This AI support system would allow for the rapid communication between operatives from different organisations without having to go up-and-down the chain of command by creating democratised information relays on every mobile device.
Challenges
While there’s a ton of technical challenges involved in creating a solution like this, we’ll leave that as a topic for another day. Today, we’d like to highlight some of the high-level challenges such a solution might face instead.
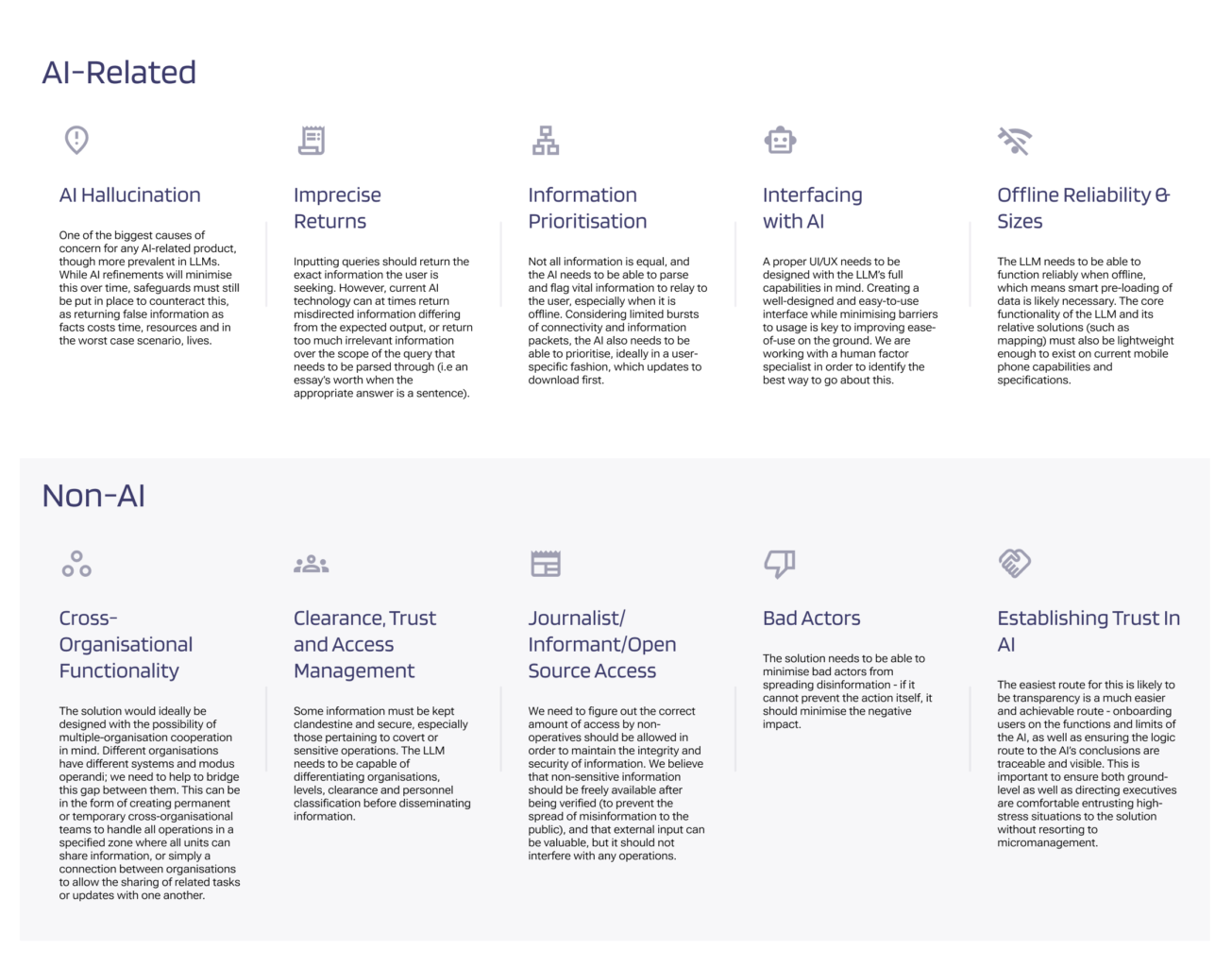
Team Learnings
From a very early stage, we latched onto the idea that information during a crisis should be democratised as much as is possible. In an emergency, manpower and time are the two most valuable resources we have, and bureaucracy and politics should play little to no part when lives are at stake. We believe that by creating an alternative solution that can direct information exactly where it needs to go ASAP, we can further improve the impact of the incredible people who work on the ground. Interestingly, something that’s become apparent to us is that the role of an AI Handler in these types of solutions is going to become a necessary component going forward - at least until AI eventually becomes reliable and safe to use for such safety-critical tasks. The specific form of handling or role this might entail is still up in the air - it might even be entirely dependent on the technology instead of the design solution. Are we looking for a database engineer of sorts? Someone familiar with the code and the workings of the AI to maintain and ensure everything is running smoothly, as well as conduct emergency maintenance by directly tinkering with the AI? Are we looking for something more akin to a dog handler? Someone trained to simply be an overwatch that helps direct the AI in the right direction and makes sure that it ‘eats healthy’. Or are we looking for a gardener? Somebody who needs to design, plan and shape the initial zone of operations into the AI and continuously prune the unnecessary bits to keep it healthy as it grows on its own? Either way, I’m excited to find out. Will keep you posted.
Mike Oliver
Easol Explores is an insight into some of the more interesting problems and projects we’ve been working on recently and a showcase of our approach to modern problems. Feel free to chime in, converse, or correct us on these topics; we’d love to discuss them further with you.